AI-Ready IOP Data: A New Lens for Understanding Glaucoma
- Tom Breunig
- 11 minutes ago
- 3 min read
As artificial intelligence (AI) begins to shape the future of medical diagnostics and personalized care, ophthalmology is poised to benefit in a uniquely data-driven way. At the center of this shift is Injectsense's IOP-Connect implantable sensor platform that generate continuous, high-fidelity intraocular pressure (IOP) data—information that is not only more comprehensive than periodic measurements but is also inherently structured for AI integration.
Technologies like IOP-Connect are producing rich, round-the-clock IOP datasets that can power machine learning algorithms, enabling a deeper understanding of glaucoma pathology and more precise, patient-specific management strategies. For clinicians, this represents more than a technological leap—it is an opportunity to practice a more proactive, data-informed form of care.
From Episodic to Continuous: Unlocking Hidden Patterns
Conventional IOP monitoring methods—whether Goldmann applanation or handheld rebound measurement devices—offer static readings during daytime hours. However, clinicians are well aware that IOP is not a static variable. Fluctuations driven by circadian rhythms, patient activity, posture, and medication timing can profoundly affect disease progression, but these patterns remain largely invisible without continuous tracking.
By contrast, implantable sensors capable of capturing minute-to-minute data transform the IOP profile from a series of snapshots into a dynamic physiological representation. This trendline is highly suitable for AI analysis, allowing models to detect outliers, uncover patterns, and correlate pressure behavior with clinical outcomes.
AI and the Real-World Physiology of IOP
The clinical potential of AI lies in its ability to sift through thousands of data points per patient to identify risk signals that human observation might miss. In glaucoma care, this could mean early identification of patients likely to progress despite so-called "normal" office IOP measurements, or predictive modeling of when pressure surges are most likely to occur.
AI systems trained on continuous IOP data can begin to define new metrics of disease control beyond single threshold values—such as pressure variability, spike frequency, or nocturnal load—and integrate these with other risk factors to improve clinical decision-making.
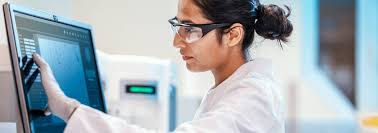
Medication Adherence and Physiologic Validation
For clinicians managing patients with poor response to topical therapy, AI-enhanced IOP monitoring offers a novel tool: real-world feedback on medication adherence. Deviations from expected pressure responses can suggest inconsistent use, guiding timely interventions or therapy adjustments.
This physiologic validation is particularly useful in differentiating non-responders from non-adherers—an important distinction that is often difficult to make using traditional methods alone.
Exploring Drug Interactions and Co-Morbid Impact
With the accumulation of continuous IOP data across diverse patient cohorts, clinicians may also begin to better understand how systemic medications, sleep patterns, and lifestyle factors influence IOP behavior. AI can play a key role in uncovering subtle correlations between polypharmacy and pressure control, which could inform treatment plans in patients with complex medical profiles.
Toward a Multimodal Understanding of Glaucoma
Perhaps most promising is the expected research on correlations between IOP data, optical coherence tomography (OCT), and Humphrey Visual Field (HVF) testing. AI models trained on multimodal data streams have the potential to map the relationship between pressure dynamics, structural changes in the optic nerve, and visual field loss over time.
This synthesis may not only enhance diagnostic accuracy but also provide new hypotheses about the mechanisms of glaucomatous damage—especially the role of pressure fluctuation as a pathogenic factor.
Clinical Pathways Forward
While these AI-enabled tools are still evolving, the foundation is being laid for a more predictive and personalized model of glaucoma care. For clinicians, engaging with continuous IOP data now may mean earlier interventions, more nuanced therapy choices, and stronger longitudinal insight into patient outcomes.
As AI systems mature alongside data acquisition platforms like IOP-Connect, ophthalmology stands at the threshold of an era where real-time physiologic data informs both individual care and the broader understanding of disease.
Comments